21. May 2025
16:00 – 16:20
AI Applications and FA Workflows
Enhancing Semiconductor Nanoprobing procedures with AI-Driven Tip Detection
David Kleindiek
Kleindiek Nanotechnik I Germany
Abstract
The automation of nanoprobing application relies on the accurate detection of probe tips in scanning electron microscope (SEM) images. This work explores the application of deep learning models to automate and improve this critical process. Different models such as Mask R-CNN, YOLOv8 and RTMDet, trained on a specialized dataset, are used to accurately detect, segment and localize probe tips in SEM images, even under challenging conditions. Results show that these models have the potential to improve the automation of nanoprobing workflows, particularly in automatic tip positioning and crash prevention. Future work will focus on production-level deployment and the integration of tracking algorithms.
Biography
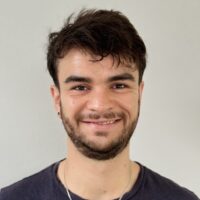
David Kleindiek is pursuing his Master’s degree in Computer Science at the University of Tübingen, specializing in Machine Learning. As a Machine Learning Engineer at Kleindiek Nanotechnik, he develops deep learning-based computer vision models to automate and optimize nanoprobing procedures in semiconductor analysis.